This AI Paper Introduces Evo: A Genomic Foundation Model that Enables Prediction and Generation Tasks from the Molecular to Genome-Scale
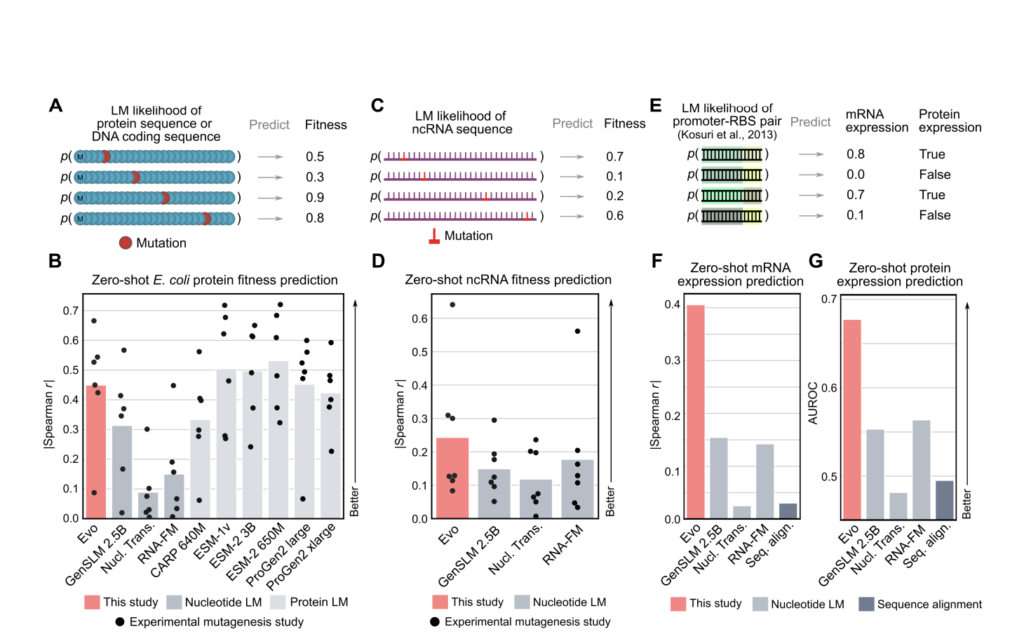
Genomic research is a critical field that focuses on understanding genomes’ structure, function, and evolution. It encompasses studies on DNA sequences, genetic variations, and the intricate mechanisms governing gene expression and regulation. This field has profound implications for biotechnology, medicine, and evolutionary biology, offering insights into genetic disorders, potential therapies, and the fundamental processes of life.
One critical problem is the need for advanced models to predict and generate biological sequences. Current methods can be more complex and scale to model genomic functions accurately. Researchers seek solutions to improve these models’ precision and efficiency to better understand and manipulate biological systems.
Current methods often need more capability to handle the complexity and scale required to model genomic functions accurately. Researchers seek solutions to improve these models’ precision and efficiency to better understand and manipulate biological systems. Traditional approaches in genomic modeling have primarily utilized modality-specific models focused on proteins, regulatory DNA, or RNA. These models often need help handling the multi-scale interactions in complex biological processes. Generative applications have been restricted to designing simple molecules and short sequences, lacking the breadth necessary for comprehensive genomic analysis.
Researchers from Stanford University, Arc Institute, TogetherAI, CZ Biohub, and the University of California, Berkeley, have introduced Evo, a genomic foundation model designed to perform prediction and generation tasks from the molecular to genome-scale. Evo leverages a novel deep signal processing architecture to handle vast genomic datasets with high precision. Evo‘s architecture incorporates a hybrid of attention mechanisms and convolutional operators, allowing it to process sequences at single-nucleotide resolution over long contexts. Trained on 7 billion parameters with data from whole prokaryotic genomes, Evo can generalize across DNA, RNA, and protein modalities, enabling it to predict gene functions and generate complex biological systems.
Evo employs a state-of-the-art deep signal processing architecture, StripedHyena, which combines attention mechanisms with convolutional operators to process long genomic sequences efficiently. This hybrid approach enables Evo to maintain high resolution at the single-nucleotide level, which is crucial for capturing the detailed variations in genetic sequences. The model is trained on extensive prokaryotic genome datasets totaling 300 billion nucleotide tokens, which include bacterial and archaeal genomes and millions of predicted phage and plasmid sequences. This comprehensive training allows Evo to learn the intricate patterns of genomic sequences, making it capable of predicting and generating tasks across different molecular modalities. The training process involved two stages: initially using a context length of 8,000 tokens and extending to 131,000 tokens to capture broader genomic contexts. Evo‘s architecture includes 29 layers of data-controlled convolutional operators interleaved with multi-head attention layers equipped with rotary position embeddings, enhancing its ability to recall long-sequence information.
The performance of Evo excels in zero-shot function prediction and generation tasks. It can generate synthetic CRISPR-Cas molecular complexes and transposable systems, predict gene essentiality with high accuracy, and create coding-rich sequences up to 650 kilobases in length. In terms of specific performance metrics, Evo demonstrated a Spearman correlation of 0.64 in predicting the fitness effects of mutations on the 5S ribosomal RNA in E. coli. For gene expression prediction, Evo achieved a correlation of 0.41 for mRNA expression and an AUROC of 0.68 for protein expression prediction. The model’s ability to predict gene essentiality was also impressive, with an AUROC of 0.86 for lambda phage essentiality and 0.81 for Pseudomonas aeruginosa. These capabilities surpass those of existing domain-specific language models, highlighting Evo‘s advanced performance across various genomic tasks. Furthermore, Evo‘s generative capabilities are demonstrated by its ability to produce coherent CRISPR-Cas systems, with 15-45% of generated sequences containing Cas coding sequences as long as 5kb and generating transposable elements with significant protein sequence diversity.
In conclusion, the research team has developed a powerful tool in Evo that addresses the limitations of previous models. By enabling comprehensive genomic analysis and generation, Evo represents a significant advancement in the field, promising to enhance our understanding and control of biological systems on multiple levels. Evo‘s success in modeling genomic data at scale and its ability to perform zero-shot predictions and generate complex biological sequences mark a significant leap forward in genomic research. This model not only provides a deeper mechanistic understanding of biology but also accelerates the potential for engineering life forms, offering a new paradigm in biological research and synthetic biology.
Check out the Paper. All credit for this research goes to the researchers of this project. Also, don’t forget to follow us on Twitter. Join our Telegram Channel, Discord Channel, and LinkedIn Group.
If you like our work, you will love our newsletter..
Don’t Forget to join our 42k+ ML SubReddit
Nikhil is an intern consultant at Marktechpost. He is pursuing an integrated dual degree in Materials at the Indian Institute of Technology, Kharagpur. Nikhil is an AI/ML enthusiast who is always researching applications in fields like biomaterials and biomedical science. With a strong background in Material Science, he is exploring new advancements and creating opportunities to contribute.