Salesforce AI Research Introduces a Novel Evaluation Framework for Retrieval-Augmented Generation (RAG) Systems based on Sub-Question Coverage
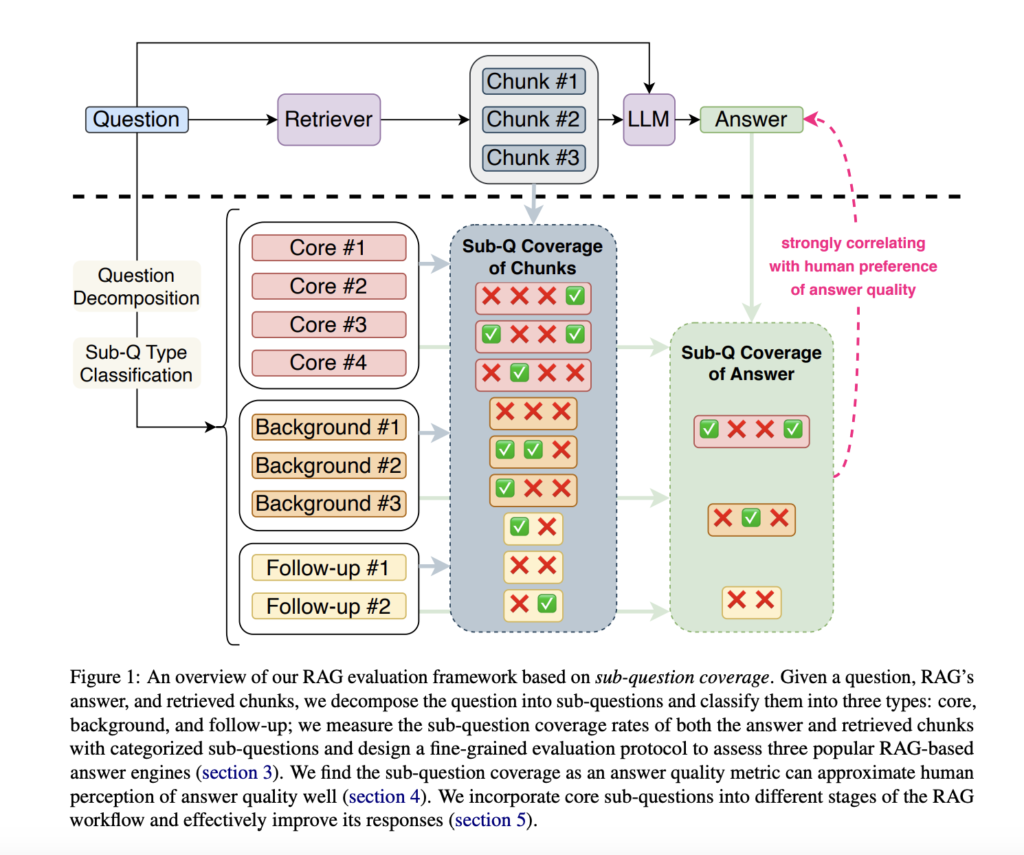
Retrieval-augmented generation (RAG) systems blend retrieval and generation processes to address the complexities of answering open-ended, multi-dimensional questions. By accessing relevant documents and knowledge, RAG-based models generate answers with additional context, offering richer insights than generative-only models. This approach is useful in fields where responses must reflect a broad knowledge base, such as legal research and academic analysis. RAG systems retrieve targeted data and assemble it into comprehensive answers, which is particularly advantageous in situations requiring diverse perspectives or deep context.
Evaluating the effectiveness of RAG systems presents unique challenges, as they often need to answer non-factoid questions that need more than a single definitive response. Traditional evaluation metrics, such as relevance and faithfulness, need to fully capture how well these systems cover such questions’ complex, multi-layered subtopics. In real-world applications, questions often comprise core inquiries supported by additional contextual or exploratory elements, forming a more holistic response. Existing tools and models focus primarily on surface-level measures, leaving a gap in understanding the completeness of RAG responses.
Most current RAG systems operate with general quality indicators that only partially address user needs for comprehensive coverage. Tools and frameworks often incorporate sub-question cues but need help to fully decompose a question into detailed sub-topics, impacting user satisfaction. Complex queries may require responses that cover not only direct answers but also background and follow-up details to achieve clarity. By needing a fine-grained coverage assessment, these systems frequently overlook or inadequately integrate essential information into their generated answers.
The Georgia Institute of Technology and Salesforce AI Research researchers introduce a new framework for evaluating RAG systems based on a metric called “sub-question coverage.” Instead of general relevance scores, the researchers propose decomposing a question into specific sub-questions, categorized as core, background, or follow-up. This approach allows a nuanced assessment of response quality by examining how well each sub-question is addressed. The team applied their framework to three widely-used RAG systems, You.com, Perplexity AI, and Bing Chat, revealing distinct patterns in handling various sub-question types. Researchers could pinpoint gaps where each system failed to deliver comprehensive answers by measuring coverage across these categories.
In developing the framework, researchers employed a two-step method as follows:
First, they broke down complex questions into sub-questions with roles categorized as core (essential to the main question), background (providing necessary context), or follow-up (non-essential but valuable for further insight).
Next, they tested how well the RAG systems retrieved relevant content for each category and how effectively it was incorporated into the final answers. For example, each system’s retrieval capabilities were examined in terms of core sub-questions, where adequate coverage often predicts the overall success of the answer.
Metrics developed through this process offer precise insights into RAG systems’ strengths and limitations, allowing for targeted improvements.
The results revealed significant trends among the systems, highlighting both strengths and limitations in their capabilities. Although each RAG system prioritized core sub-questions, none achieved full coverage, with gaps remaining even in critical areas. In You.com, the core sub-question coverage was 42%, while Perplexity AI performed better, reaching 54% coverage. Bing Chat displayed a slightly lower rate at 49%, although it excelled in organizing information coherently. However, the coverage for background sub-questions was notably low across all systems, 20% for You.com and Perplexity AI and only 14% for Bing Chat. This disparity reveals that while core content is prioritized, systems often need to pay more attention to supplementary information, impacting the response quality perceived by users. Also, researchers noted that Perplexity AI excelled in connecting retrieval and generation stages, achieving 71% accuracy in aligning core sub-questions, whereas You.com lagged at 51%.
This study highlights that evaluating RAG systems requires a shift from conventional methods to sub-question-oriented metrics that assess retrieval accuracy and response quality. By integrating sub-question classification into RAG processes, the framework helps bridge gaps in existing systems, enhancing their ability to produce well-rounded responses. Results show that leveraging core sub-questions in retrieval can substantially elevate response quality, with Perplexity AI demonstrating a 74% win rate over a baseline that excluded sub-questions. Importantly, the study identified areas for improvement, such as Bing Chat’s need to increase the coherence of core-to-background information alignment.
Key takeaways from this research underscore the importance of sub-question classification for improving RAG performance:
Core Sub-question Coverage: On average, RAG systems missed around 50% of core sub-questions, indicating a clear area for improvement.
System Accuracy: Perplexity AI led with a 71% accuracy in connecting retrieved content to responses, compared to You.com’s 51% and Bing Chat’s 63%.
Importance of Background Information: Background sub-question coverage was lower across all systems, ranging between 14% and 20%, suggesting a gap in contextual support for responses.
Performance Rankings: Perplexity AI ranked highest overall, with Bing Chat excelling in structuring responses and You.com showing notable limitations.
Potential for Improvement: All RAG systems showed substantial room for enhancement in core sub-question retrieval, with projected gains in response quality as high as 45%.

In conclusion, this research redefines how RAG systems are assessed, emphasizing sub-question coverage as a primary success metric. By analyzing specific sub-question types within answers, the study sheds light on the limitations of current RAG frameworks and offers a pathway for enhancing answer quality. The findings highlight the need for focused retrieval augmentation and point to practical steps that could make RAG systems more robust for complex, knowledge-intensive tasks. The research sets a foundation for future improvements in response generation technology through this nuanced evaluation approach.
Check out the Paper. All credit for this research goes to the researchers of this project. Also, don’t forget to follow us on Twitter and join our Telegram Channel and LinkedIn Group. If you like our work, you will love our newsletter.. Don’t Forget to join our 55k+ ML SubReddit.
[Upcoming Live Webinar- Oct 29, 2024] The Best Platform for Serving Fine-Tuned Models: Predibase Inference Engine (Promoted)
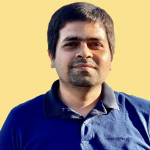
Asif Razzaq is the CEO of Marktechpost Media Inc.. As a visionary entrepreneur and engineer, Asif is committed to harnessing the potential of Artificial Intelligence for social good. His most recent endeavor is the launch of an Artificial Intelligence Media Platform, Marktechpost, which stands out for its in-depth coverage of machine learning and deep learning news that is both technically sound and easily understandable by a wide audience. The platform boasts of over 2 million monthly views, illustrating its popularity among audiences.