Rethinking Direct Alignment: Balancing Likelihood and Diversity for Better Model Performance
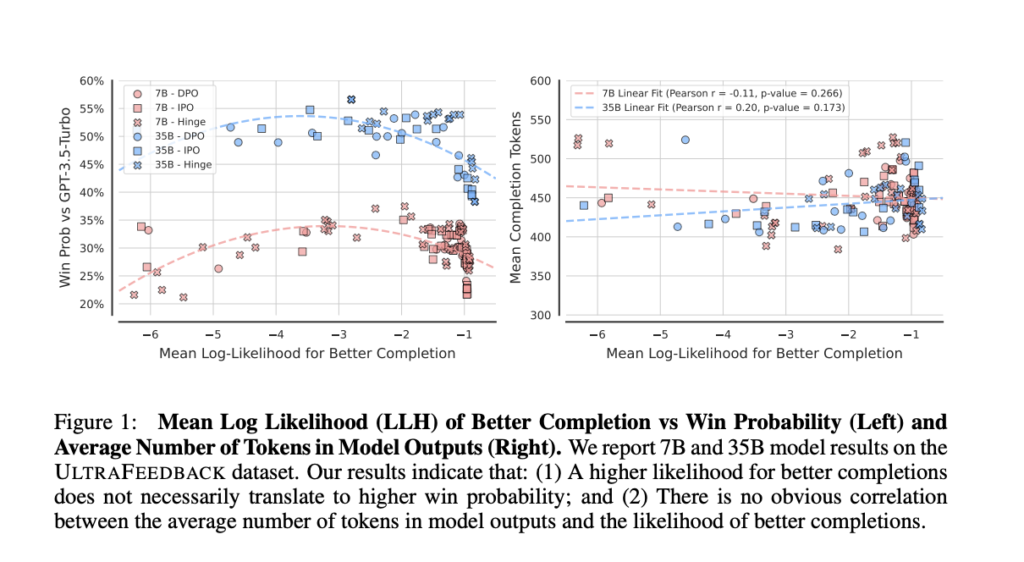
The problem of over-optimization of likelihood in Direct Alignment Algorithms (DAAs), such as Direct Preference Optimisation (DPO) and Identity Preference Optimisation (IPO), arises when these methods fail to improve model performance despite increasing the likelihood of preferred outcomes. These algorithms, which are alternatives to Reinforcement Learning from Human Feedback (RLHF), aim to align language models with human preferences by directly optimizing for desired outcomes without explicit reward modeling. However, optimizing likelihood alone can sometimes degrade model performance, indicating a fundamental flaw in using likelihood as the primary alignment objective.
Researchers from University College London and Cohere explore the issue of likelihood over-optimization in state-of-the-art Direct Alignment Algorithms DAAs, investigating whether increasing the likelihood of better (i.e., preferred) completions and minimizing the likelihood of worse completions leads to improved performance. The study reveals that higher likelihood does not always correspond with better model performance, particularly in terms of alignment with human preferences. Instead, they find that slightly lowering the likelihood tends to enhance the diversity of model outputs, which improves generalization to unseen data. Furthermore, the researchers identify two key indicators that signal when over-optimization begins to degrade performance: decreasing entropy over Top-k Tokens and diminishing Top-k Probability Mass.
The structure of this research approach includes an in-depth analysis of the relationship between completion likelihood and performance metrics across different DAAs. The researchers utilized two instruction-tuned models (7B and 35B parameters) trained on the ULTRAFEEDBACK dataset, which contains binarized preference data. They trained each model using different hyperparameters for DPO, IPO, and a Hinge loss function, monitoring the log-likelihood of preferred completions. The study also employed regularization schemes like Negative Log-Likelihood (NLL) to mitigate over-optimization and evaluated generalization performance using LLM-as-a-Judge, a framework for comparing model outputs with those from other leading models.
The experimental results showed that higher likelihoods of preferred completions do not necessarily improve win probability when compared to models like GPT-3.5 Turbo. For instance, both 7B and 35B models showed weak correlations between completion likelihood and improved win probability, suggesting that an overly high completion likelihood can actually harm model performance. Furthermore, models with a slightly reduced likelihood of preferred completions tended to exhibit greater output diversity, which correlated positively with improved generalization. This improvement was particularly significant during the early stages of training. Importantly, the study outlined how excessive diversity, although beneficial initially, could eventually degrade model performance if the model starts generating overly random outputs.
The conclusion of the research emphasizes that maintaining an optimal balance between increasing the likelihood of preferred completions and promoting diversity is critical for improving model performance. The researchers propose monitoring entropy and probability mass as early indicators of over-optimization to prevent performance decline. They also suggest that adaptive regularization techniques could be employed during training to achieve this balance. The implications of these findings are significant for enhancing offline preference learning methods, offering strategies to optimize DAAs without falling into the trap of over-optimization.
Check out the Paper. All credit for this research goes to the researchers of this project. Also, don’t forget to follow us on Twitter and join our Telegram Channel and LinkedIn Group. If you like our work, you will love our newsletter.. Don’t Forget to join our 50k+ ML SubReddit.
[Upcoming Live Webinar- Oct 29, 2024] The Best Platform for Serving Fine-Tuned Models: Predibase Inference Engine (Promoted)
Asif Razzaq is the CEO of Marktechpost Media Inc.. As a visionary entrepreneur and engineer, Asif is committed to harnessing the potential of Artificial Intelligence for social good. His most recent endeavor is the launch of an Artificial Intelligence Media Platform, Marktechpost, which stands out for its in-depth coverage of machine learning and deep learning news that is both technically sound and easily understandable by a wide audience. The platform boasts of over 2 million monthly views, illustrating its popularity among audiences.