NVIDIA AI Introduces Nemotron-4 340B: A Family of Open Models that Developers can Use to Generate Synthetic Data for Training Large Language Models (LLMs)
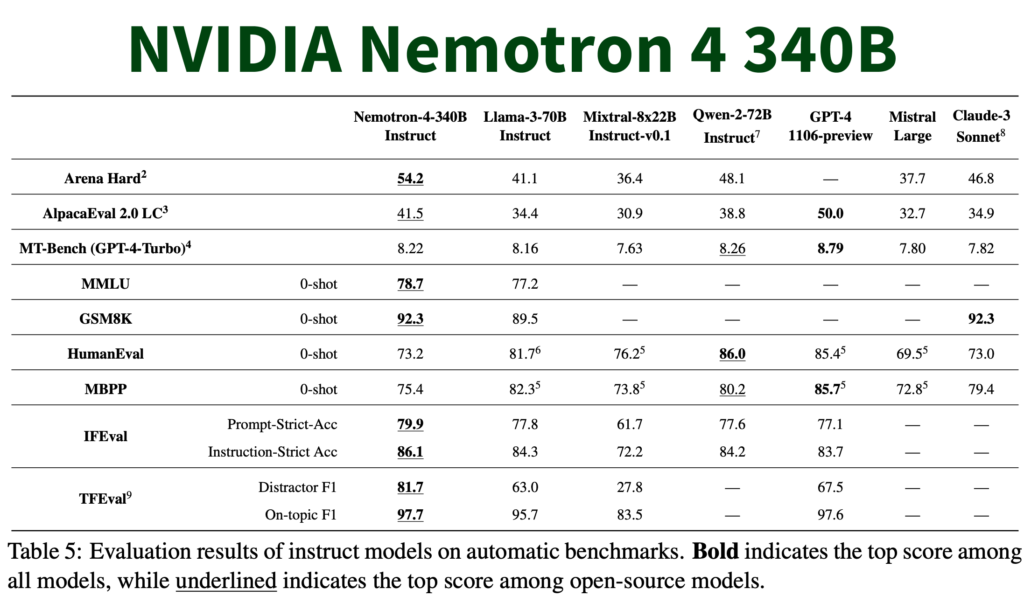
NVIDIA has recently unveiled the Nemotron-4 340B, a groundbreaking family of models designed to generate synthetic data for training large language models (LLMs) across various commercial applications. This release marks a significant advancement in generative AI, offering a comprehensive suite of tools optimized for NVIDIA NeMo and NVIDIA TensorRT-LLM and includes cutting-edge instruct and reward models. This initiative aims to provide developers with a cost-effective and scalable means to access high-quality training data, which is crucial for enhancing the performance and accuracy of custom LLMs. The Nemotron-4 340B includes three variants: Instruct, Reward, and Base models, each tailored to specific functions in the data generation and refinement process.
The Nemotron-4 340B Instruct model is designed to create diverse synthetic data that mimics the characteristics of real-world data, enhancing the performance and robustness of custom LLMs across various domains. This model is essential for generating initial data outputs, which can be refined and improved upon.
The Nemotron-4 340B Reward model is crucial in filtering and enhancing the quality of AI-generated data. It evaluates responses based on helpfulness, correctness, coherence, complexity, and verbosity. This model ensures that the synthetic data is high quality and relevant to the application’s needs.
The Nemotron-4 340B Base model serves as the foundational framework for customization. Trained on 9 trillion tokens, this model can be fine-tuned using proprietary data and various datasets to adapt to specific use cases. It supports extensive customization through the NeMo framework, allowing for supervised fine-tuning and parameter-efficient methods like low-rank adaptation (LoRA).
This innovative model family boasts impressive specifications, including a 4k context window, training in over 50 and 40 programming languages, and achieving notable benchmarks such as 81.1 MMLU, 90.53 HellaSwag, and 85.44 BHH. The models require significant computational power, including 16x H100 GPUs in bf16 and approximately 8x H100 in int4 configurations.
High-quality training data is important for developing robust LLMs but often comes with substantial costs and accessibility issues. Nemotron-4 340B addresses this challenge by enabling synthetic data generation through a permissive open model license. This model family includes base, instruct, and reward models, forming a pipeline that facilitates the creation and refinement of synthetic data. These models are seamlessly integrated with NVIDIA NeMo, an open-source framework that supports end-to-end model training, encompassing data curation, customization, and evaluation. They are optimized for inference using the NVIDIA TensorRT-LLM library, enhancing their efficiency and scalability.
The Nemotron-4 340B Instruct model is particularly noteworthy as it generates synthetic data that closely mimics real-world data, improving the data quality and enhancing the performance of custom LLMs across diverse domains. This model can create varied and realistic data outputs, which can then be refined using the Nemotron-4 340B Reward model. The Reward model evaluates responses based on helpfulness, correctness, coherence, complexity, and verbosity, ensuring the generated data meets high-quality standards. This evaluation process is critical for maintaining the relevance and accuracy of synthetic data, making it suitable for various applications.
One of Nemotron-4 340 B’s key advantages is its customization capabilities. Researchers and developers can tailor the base model using proprietary data, including the HelpSteer2 dataset, allowing for creating bespoke instruct or reward models. This customization process is facilitated by the NeMo framework, which supports various fine-tuning methods, including supervised fine-tuning and parameter-efficient approaches like LoRA. These methods enable developers to adapt the models to specific use cases, improving their accuracy and effectiveness in downstream tasks.
The models are optimized with TensorRT-LLM to leverage tensor parallelism, a form of model parallelism that distributes individual weight matrices across multiple GPUs and servers. This optimization allows for efficient inference at scale, making it possible to handle large datasets and complex computations more effectively.
The release of Nemotron-4 340B also emphasizes the importance of model security and evaluation. The Instruct model underwent rigorous safety evaluations, including adversarial testing, to ensure reliability across various risk indicators. Despite these precautions, NVIDIA advises users to evaluate the model outputs thoroughly to ensure the synthetic data generated is safe, accurate, and suitable for their specific use cases.
Developers can access the Nemotron-4 340B models on platforms like Hugging Face, and they will soon be available as an NVIDIA NIM microservice with a standard API. This accessibility, combined with the models’ robust capabilities, positions Nemotron-4 340B as a valuable tool for organizations seeking to harness the power of synthetic data in their AI development processes.
In conclusion, NVIDIA’s Nemotron-4 340B represents a leap forward in generating synthetic data for training LLMs. Its open model license, advanced instruct and reward models, and seamless integration with NVIDIA’s NeMo and TensorRT-LLM frameworks provide developers with powerful tools to create high-quality training data. This innovation is set to drive advancements in AI across many industries, from healthcare to finance and beyond, enabling the development of more accurate and effective language models.
Check out the Technical Report, Blog, and Models. All credit for this research goes to the researchers of this project. Also, don’t forget to follow us on Twitter.
Join our Telegram Channel and LinkedIn Group.
If you like our work, you will love our newsletter..
Don’t Forget to join our 44k+ ML SubReddit
Asif Razzaq is the CEO of Marktechpost Media Inc.. As a visionary entrepreneur and engineer, Asif is committed to harnessing the potential of Artificial Intelligence for social good. His most recent endeavor is the launch of an Artificial Intelligence Media Platform, Marktechpost, which stands out for its in-depth coverage of machine learning and deep learning news that is both technically sound and easily understandable by a wide audience. The platform boasts of over 2 million monthly views, illustrating its popularity among audiences.