MOSEL: Collection of Open Source Speech Data for Speech Foundation Model Training on EU Languages
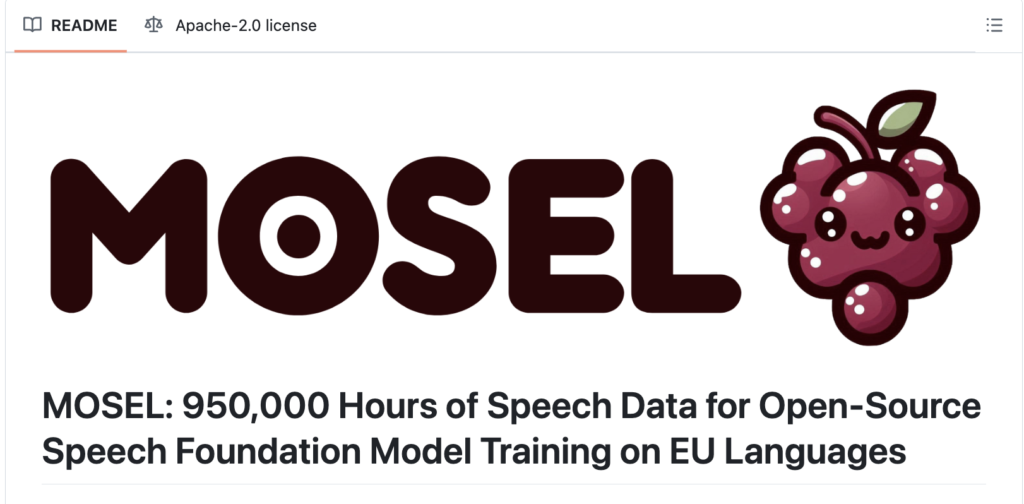
While existing speech datasets are heavily skewed towards English, many EU languages are underserved in terms of accessible and high-quality speech data. This lack of resources leads to AI models that better understand and process English than other languages in tasks like recognition, machine translation, and other natural language processing tasks. The scarcity of well-organized, large-scale, open-source datasets for EU languages leads to language bias, reduced accuracy, and limited access to AI technologies for speakers of non-English EU languages. While there are efforts to collect speech data for minority languages, they tend to be fragmented or insufficient for training foundation models on a large scale
To address this challenge, researchers introduced Mosel, a collection of open-source speech data, which offers a comprehensive solution by creating an extensive, open-source speech dataset specifically designed for EU languages. The dataset, consisting of over 950,000 hours of speech data across 24 languages, is a significant step towards reducing language bias in AI models. Mosel provides a structured, multilingual resource that addresses the gap in available data for EU languages, thereby supporting the development of more accurate and fair language models.
The Mosel dataset is built through a multi-faceted data collection, processing, and annotation approach. The project aggregates speech data from diverse sources, including public domain recordings and licensed datasets, ensuring broad language representation. Each dataset is rigorously cleaned and processed to remove inconsistencies, making it suitable for machine-learning applications. Annotations such as transcriptions, speaker metadata, and language labels are added to enhance the usability of the dataset for various AI tasks.
Mosel’s open-source licensing ensures that the dataset is freely available to researchers and developers, facilitating wide-scale use and reuse. Its architecture is designed to handle efficient data management and access, supporting tasks like data exploration and retrieval. When trained on Mosel’s dataset, the AI model’s performance is expected to improve significantly, with better accuracy in speech recognition, translation, and other natural language processing tasks. By providing a large-scale, well-annotated resource, Mosel helps models learn more nuanced linguistic patterns and reduces the bias that typically favors English.
In conclusion, the Mosel dataset represents a crucial advancement in addressing the shortage of open-source speech data for EU languages. Offering a large, diverse, and accessible corpus enables the training of more accurate and less biased AI models. This project not only enhances language-specific capabilities for EU languages but also promotes inclusive research and innovation in AI technologies across Europe.
Check out the GitHub. All credit for this research goes to the researchers of this project. Also, don’t forget to follow us on Twitter and join our Telegram Channel and LinkedIn Group. If you like our work, you will love our newsletter.. Don’t Forget to join our 50k+ ML SubReddit
Interested in promoting your company, product, service, or event to over 1 Million AI developers and researchers? Let’s collaborate!
Pragati Jhunjhunwala is a consulting intern at MarktechPost. She is currently pursuing her B.Tech from the Indian Institute of Technology(IIT), Kharagpur. She is a tech enthusiast and has a keen interest in the scope of software and data science applications. She is always reading about the developments in different field of AI and ML.